

Specifically, we found that the center point and vertices of a cell have a mutual-directed relationship that can be used to group the cells into tables by using the common vertex that is located in the intersect of the adjacent cells. It simultaneously detects the vertices and center points of tabular cells, and groups the cells into tables by learning the common vertices.

With our proposed WTW dataset available, we address the problem of table structure parsing in the wild by proposing a simple yet effective approach Cycle-CenterNet. However, limited by the training datasets used for table structure parsing, they still addressed this problem under the well-aligned assumption of tabular images. Recently, deep learning-based approaches are presented to avoid the heuristic grouping scheme design and resort to developing end-to-end models. , tackle the TSP problem in a bottom-up manner by heuristically grouping detected cells based on low-level cues (, lines, boundaries and word regions). In such scenario, the tabular images are taken with well imaging conditions and are often horizontally (or vertically) aligned with clean background and clear table structures.Įarly pioneering works, Previously, this problem is studied as table structure recognition focusing on document images. Given an image, Table Structure Parsing (TSP) aims at extracting all the tables, locating their cells, and obtaining the row-column information in the image. A more comprehensive experimentalĪnalysis also validates the advantages of our proposed methods for the TSP Improvement evaluated by the TEDS metric. Of table structure parsing on the new WTW dataset by 24.6% absolute We demonstrate that our Cycle-CenterNet consistently achieves the best accuracy Scenes like the photo, scanning files, web pages, etc. Includes well-annotated structure parsing of multiple style tables in several Present a large-scale dataset, named Wired Table in the Wild (WTW), which
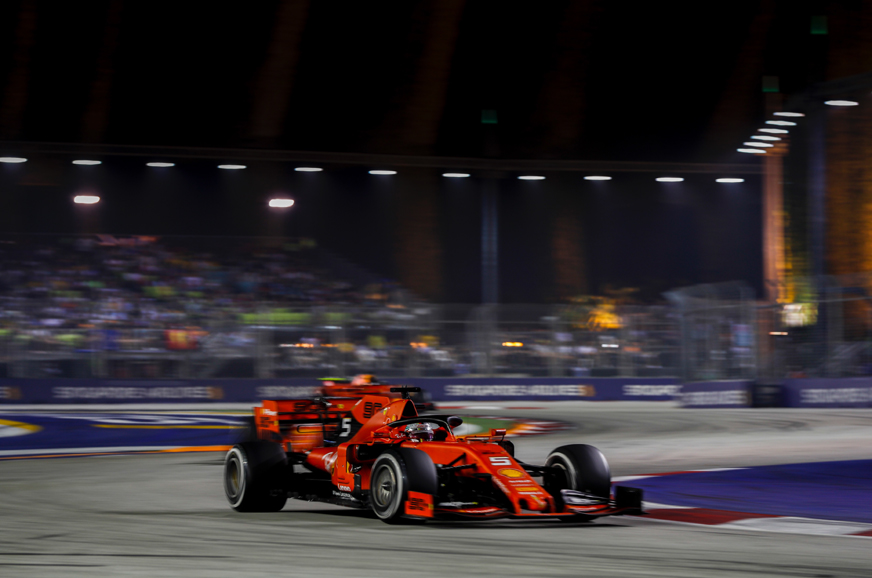
Alongside with our Cycle-CenterNet, we also In the cycle-pairing module, a new pairing loss function is For designing such a system, we propose anĪpproach named Cycle-CenterNet on the top of CenterNet with a novelĬycle-pairing module to simultaneously detect and group tabular cells into Scenarios where tabular input images are taken or scanned with severeĭeformation, bending or occlusions.
0p f1 2019 image pdf#
Well-aligned tabular images with simple layouts from scanned PDF documents, weĪim to establish a practical table structure parsing system for real-world In contrast to existing studies that mainly focus on parsing This paper tackles the problem of table structure parsing (TSP) from images
